
It was the Chinese philosopher Confucius who said, “Life is really simple, but we insist on making it complicated.”
When it comes to training artificial intelligence systems, AI engineers are discovering that the complexities of human behavior are demanding new approaches towards teaching AI to understand us.
A recent study by a team of AI and machine learning specialists at Google has exposed the problem of ‘underspecification’ as one of the biggest threats to creating accurate and reliable AI systems, a problem, which if not overcome, may well severely damage the reputation of AI and hinder its mass adoption.
What is Underspecification?
Underspecification is the phenomena by which certain elements of a system’s broader range of tasks or usage requirements are not adequately reflected in the teaching approach and testing models used to create it.
By failing to create a comprehensive training specification that accounts for the system’s interpretation of minor data points that might not seem significant in small scale lab tests but which become significant in large scale use, AI engineers have been creating AI systems that are not up to the task they are designed for.
The critical nature of this problem is manifested in the fact that underspecification often results in identical but separately trained AI systems, producing quite different results when applied to large scale data analysis tasks, despite using identical data pools and having passed lab testing.
This happens because each separate system has learned to react to its machine learning training in a slightly different way. Even if the training data set and parameters are the same, should just a single node of one AI system find itself with a different value, this will result in the analyzed data being represented or interpreted in a different way when scaled up. While this might go unnoticed in a small-scale lab test, this single difference might have huge consequences in large scale mass use.
To put this problem into context, imagine that a hedge fund manager is about to invest billions of dollars of his client’s money into the stock market, only to get conflicting information from the two AI investment platforms they are using. Such an outcome could result in the trader losing substantial sums of money. This scenario is one possible result of underspecification.
Unfortunately, underspecification is not a problem that can easily be overcome as it demands that engineers develop new training approaches and use larger pools of data in order to teach their systems to be more accurate when using large data pools. To make the problem even more complex, Google’s study leader, Alex D’Amour, highlighted that this phenomenon “happens all over the place,” and so can be overlooked even by large scale training models.
This is a daunting realization for all AI developers, particularly when these systems are trying to understand something as diverse as human behavior.
Read Also
- When We See The World’s First AI Movie Director?
- Does Big Data Analytics Have The Power To Change How Movie Theatres Do Business?
Underspecification in AI-assisted Moviemaking
The seriousness of Google’s findings will likely affect every segment of the artificial intelligence industry.
The result is that the industries that rely most heavily on AI such as finance, healthcare, and chatbots are certain to demand AI companies enact changes to their existing AI training programs, other smaller AI segments such as AI-assisted moviemaking are likely to not be far behind.
It is important to note that AI-aided moviemaking platforms are already achieving very respectable degrees of accuracy when it comes to key areas of development. Several of the top AI-assisted filmmaking platforms have already reached accuracy milestones that have convinced several major Hollywood film studios to invest in their services. One example is ‘Gross earning predictions’, which allow production companies to gain accurate insights into the potential box office earnings of their projects. Leading AI companies have already succeeded in achieving accuracy rates of 86% in this area.
However, it is likely that underspecification represented a factor that initially contributed to lower accuracy levels when these systems were first rolled out. Going forward, problems overlooked as a result of underspecification might well need to be identified and ‘retrained’ in order for these AI moviemaking systems to push their prediction accuracy levels higher.
It is likely that underspecification in AI moviemaking solutions will have a bigger impact when it comes to issues such as identification and equality. Given that gender identification, for example, has only recently been partially freed from ‘the closet’, by which we mean that individuals are finally becoming free to define their own genders, it seems certain that it is going to take some time until all forms of gender and how they choose to be represented can be mapped by AI engineers.
Naturally, this poses a huge problem for AI moviemaking technicians in creating comprehensive AI training programs that will be able to perform such actions as identifying different genders and prejudicial representations or bias towards them onscreen.
At least one AI moviemaking company has focused the mainstay of its efforts on a mission to combat inequality in film. Without a broad understanding of these emerging genders, however, it seems inevitable that its platform will, at least for some time, exhibit gender-based biases resulting from the basic lack of understanding that has fueled this problem in the first place.
The AI companies that do push ahead with such approaches run a very real and dangerous risk of their systems, suffering from underspecification problems, extrapolating patterns or results that exhibit blatant offensive biases that enrage certain minorities and cause a tidal wave of damaging press coverage that services to irreversibly damage their reputation.
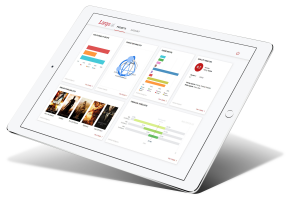
It is clear that AI companies in all industries must proceed with extreme caution, particularly in industries where offending people could result in a doomed company image. Given that many of the latest AI systems are trying to ‘understand’ our wide range of human behaviors their engineers are going to have to pay more attention to underspecification.
Part of the problem to overcome underspecification lies in improving the training process. As Google’s study head D’Amour put it, “we need to get better at specifying exactly what our requirements are for our models because often what ends up happening is that we discover these requirements only after the model has failed out in the real world.”
Read Also
- How Artificial Intelligence Is Being Used to Maximize Profitability During Post-Production
- How Actors Will Benefit from Using AI: Artificial Intelligence in Filmindustry

Stay connected