
Without good data, there can be no good artificial intelligence.
Just as we humans require data to make our decisions, AI systems do also. In the same way, the quality of the data that we have access to influences the quality of the decisions that both AI and humans make too. In order for both us and AI systems to make the best decisions, the quality of the data must be of the best quality possible.
The problem is that quite often poor quality data gets mixed in with the good. This can sometimes have a big impact on the accuracy of the decisions or insights provided by the AI system. No matter what the AI system is designed to do, accuracy is key to its success.
What exactly do we mean by poor quality data vs good quality data? Well, imagine that you were training an AI system to identify the difference between a good film and a bad one. Now, naturally, there are a huge number of variables here. Age, cultural background, interests, etc., all have an impact on each individual’s decision. So for the sake of clarity, let’s reduce the variables to highlight what we mean.
Let’s see how the quality of the data would impact an AI system trying to learn what films a 12-year-old girl would think would be good and what films would be bad. What kinds of data would be useful for the system?
The most obvious example of good data would be past films loved by 12-year-old girls. However, perhaps surprisingly to non-big data specialists, a good data set example would also be films of similar genre that were not liked by 12-year-old girls. These allow the system to gain a better sense of perspective over the ‘why’ behind what 12-year-old girls like and dislike.
In the above scenario, an example of bad quality data would be a data pool that involved past generations of children and their likes and dislikes. So, by only providing the system data relating to the 1950s, for example, the system would be able to learn to understand what is popular for that generation but would likely totally fail when it came to predicting films that today’s 12-year-old girls would like.
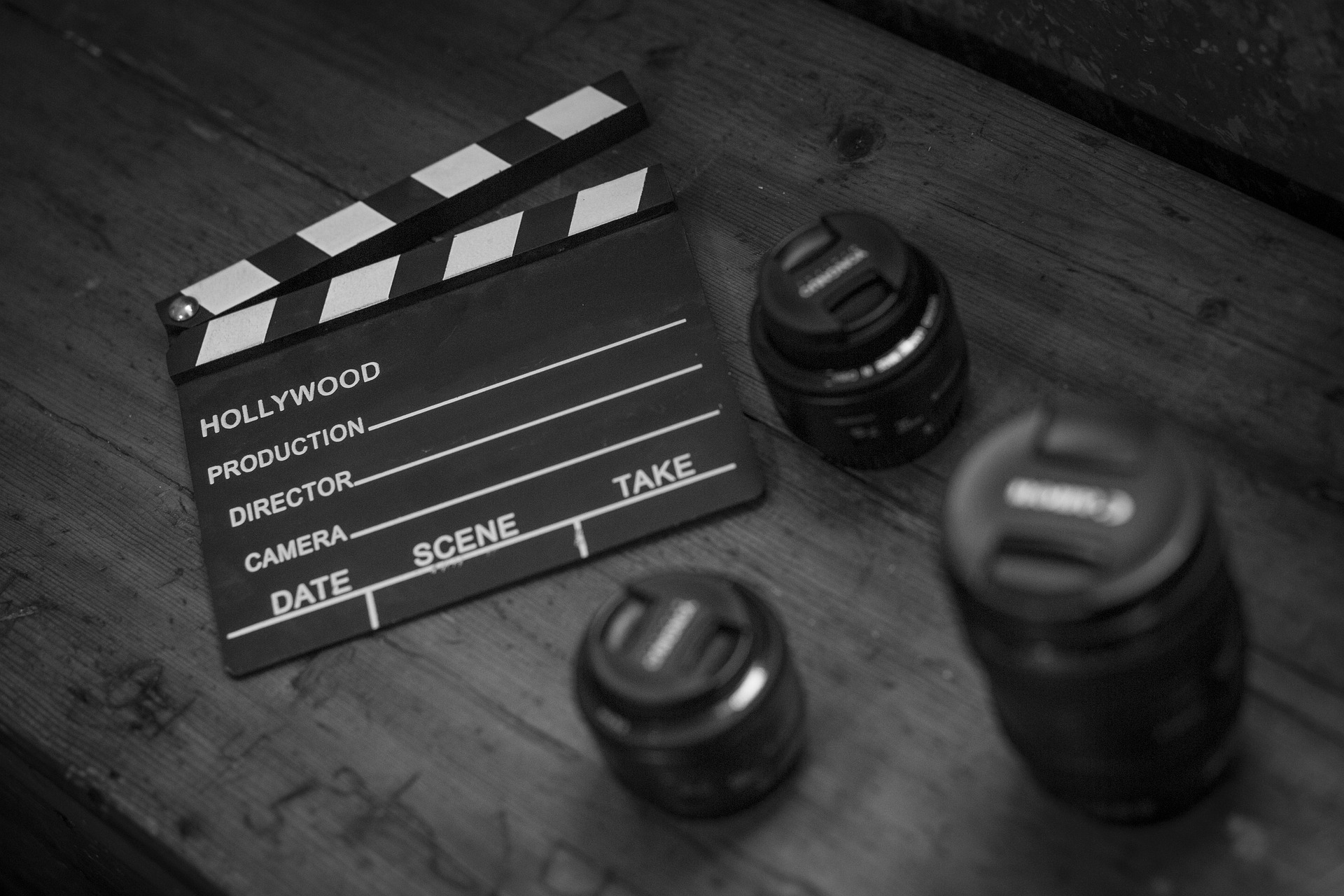
When you consider the complexity of global demographics and our different cultural backgrounds, and how quickly these are changing, the enormity of the task of training an AI system to predict which films an ever-changing range of 12-year-old girls will like becomes apparent.
While the world’s leading AI assisted filmmaking companies have achieved accuracy levels of 70%+ when making predictions as to which films will be popular and which won’t, the above example highlights that unless a solution is found, these companies will have to dedicate an enormous amount of resources to train their systems to ignore bad data and focus on good data that will allow it to keep up with the latest trends, particularly when it comes to young and upcoming generations.
Fortunately, a team of researchers and data scientists at the MIT-IBM Watson AI Lab might have just discovered a path to overcoming the problem that shifts or anomalies in new data pools present to AI systems.
Though working on a completely different problem, namely, trying to develop an AI system that was able to monitor an electricity grid in order to quickly identify if and where sources of power disruption would occur, the team managed to demonstrate that “their artificial intelligence method, which learns to model the interconnectedness of the power grid, is much better at detecting these glitches than some other popular techniques.” Source MIT News
But how can a system that is designed to detect anomalies in an electricity power grid help AI to improve the filmmaking process?

Well, the answer is in the nature of the system itself.
“To learn the complex conditional probability distribution of the data, the researchers used a special type of deep-learning model called a normalizing flow, which is particularly effective at estimating the probability density of a sample.” Source MIT News
The underlying focus of their research was on the understanding of the conditional relationships identified by the AI system between all the sensors in the electricity network. Since the sensors of the system are interacting with each other, the system is able to use a normalizing flow model using a type of graph, known as a Bayesian network, to break down the multiple time series data into less complex, conditional probabilities that are much easier to parameterize, learn, and evaluate. From this data, the AI system can help data scientists understand the probability of certain events such as a power surge occuring.
“Their model outperformed all the baselines by detecting a higher percentage of true anomalies in each dataset.” Source MIT News
The reason their model has such profound implications is that it is extremely flexible and can be used for a wide range of applications that involve large unstructured data sets. While their model was used to predict patterns or the likelihood of anomalies in a system using sensor data, it could eventually be developed into a system that was able to identify and predict changing trends such as film viewing tastes.
With sufficient development, and with sufficient ‘good’ real-time data sources, the system could learn to identify emerging cultural trends. A good example of this can be seen with the Lord of the Rings franchise, and how it popularized the, until that time, marginal Fantasy film genre and turn it into a mainstream genre. Today, countless shows and films like Game of Thrones owe an enormous debt of gratitude to the Lord of the Rings trilogy for opening up the floodgates.
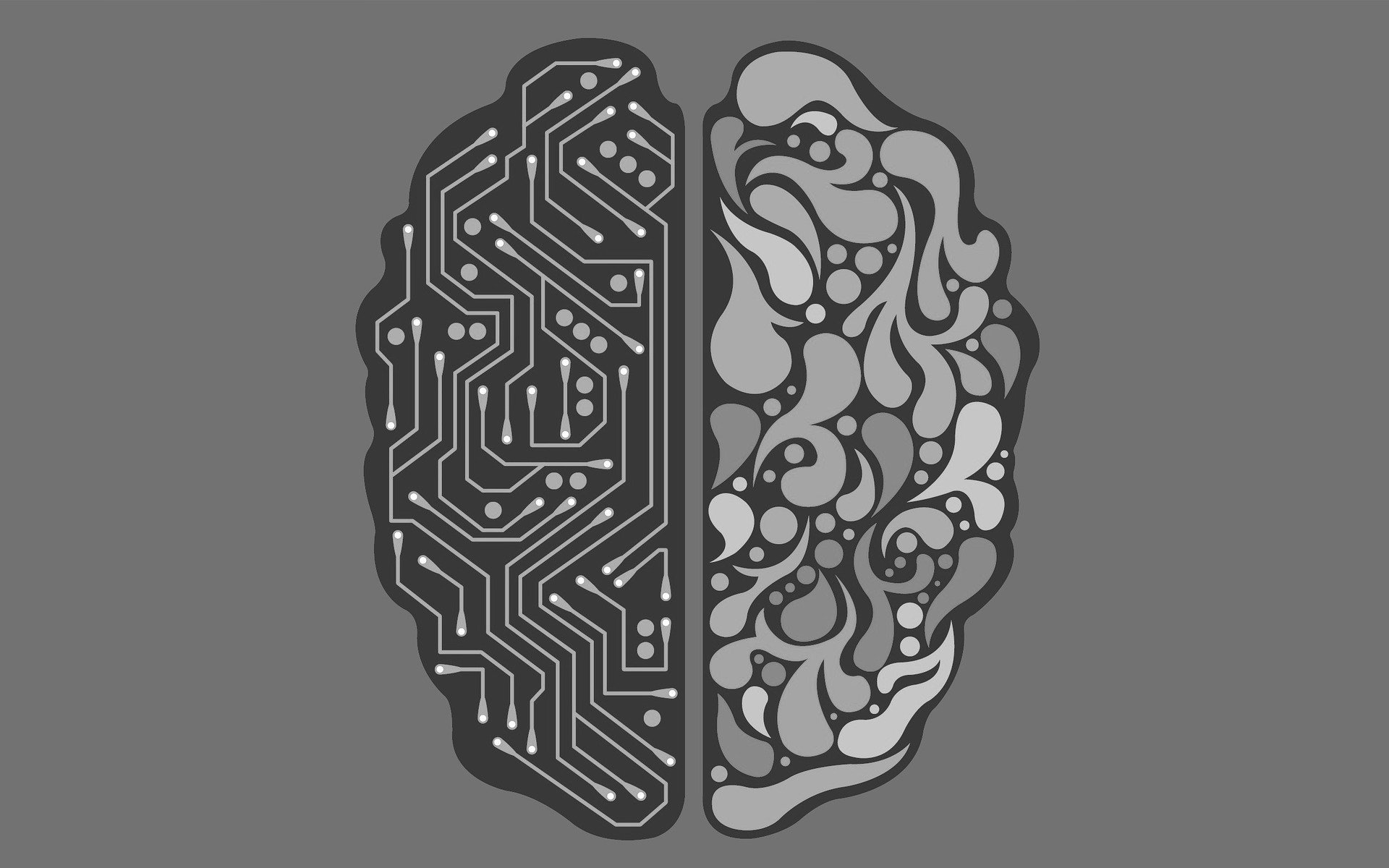
But how could an AI film system help identify cultural changes?
If we were to consider each human and their digital interactions via social media, their likes, YouTube viewing patterns, etc as an individual sensor, the AI system might be able to make predictions as to future trends in real-time and therefore provide filmmakers and producers accurate data on upcoming markets.
One obvious example would be an AI system with access to YouTube viewing trends. Such a system would be able to identify that a particular type of video, theme of content, etc., was trending and would be able to give demographic appeal data relating to this trend. Imagine the power of the system to use multiple data sources such as fashion trends to trending books, all of this information would allow the system to accurately predict future trends that would allow producers to understand which films to green light.
Such a glimpse into future trends would be a game-changer for the global film industry and could guarantee massive financial rewards. With the help of AI, it might be possible to start making the next ‘Generation movies’ just in time for the upcoming generation to be ready to view them.

Stay connected